Classification Matters: Improving Video Action Detection with Class-Specific Attention
Jul 1, 2024·
,,,,,,·
0 min read

Jinsung Lee
Taeoh Kim
Inwoong Lee
Minho Shim
Dongyoon Wee
Minsu Cho
Suha Kwak
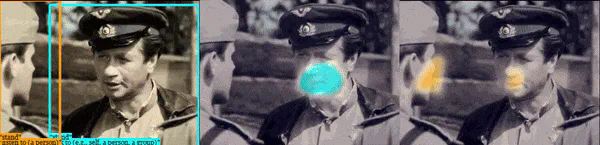
Abstract
Video action detection (VAD) aims to detect actors and classify their actions in a video. We figure that VAD suffers more from classification rather than localization of actors. Hence, we analyze how prevailing methods form features for classification and find that they prioritize actor regions for classification, yet often overlooking the essential contextual information necessary for accurate classification. Accordingly, we propose to reduce the model’s bias toward the actor itself and encourage it to pay attention to the context that is relevant to each action class. By assigning a class-dedicated query to each action class, the model can dynamically determine where to focus for effective classification. The proposed method demonstrates superior performance on three challenging benchmarks while using significantly fewer parameters and less computation.
Type
Publication
In European Conference on Computer Vision 2024 (oral)